Improving battery longevity & safety with explainable ML
In collaboration with Prof. Guozhong Cao’s group from UW’s Materials Science and Engineering department, the Battery Intelligence Group developed an explainable machine learning algorithm, “Plum,” to decouple the collective effects of cycling conditions on battery aging. Using explainable ML strategies enabled us to identify feature contributions and interactions that could minimize battery degradation at different states of health (SOH), ultimately leading to the design of safer and longer-lasting batteries.
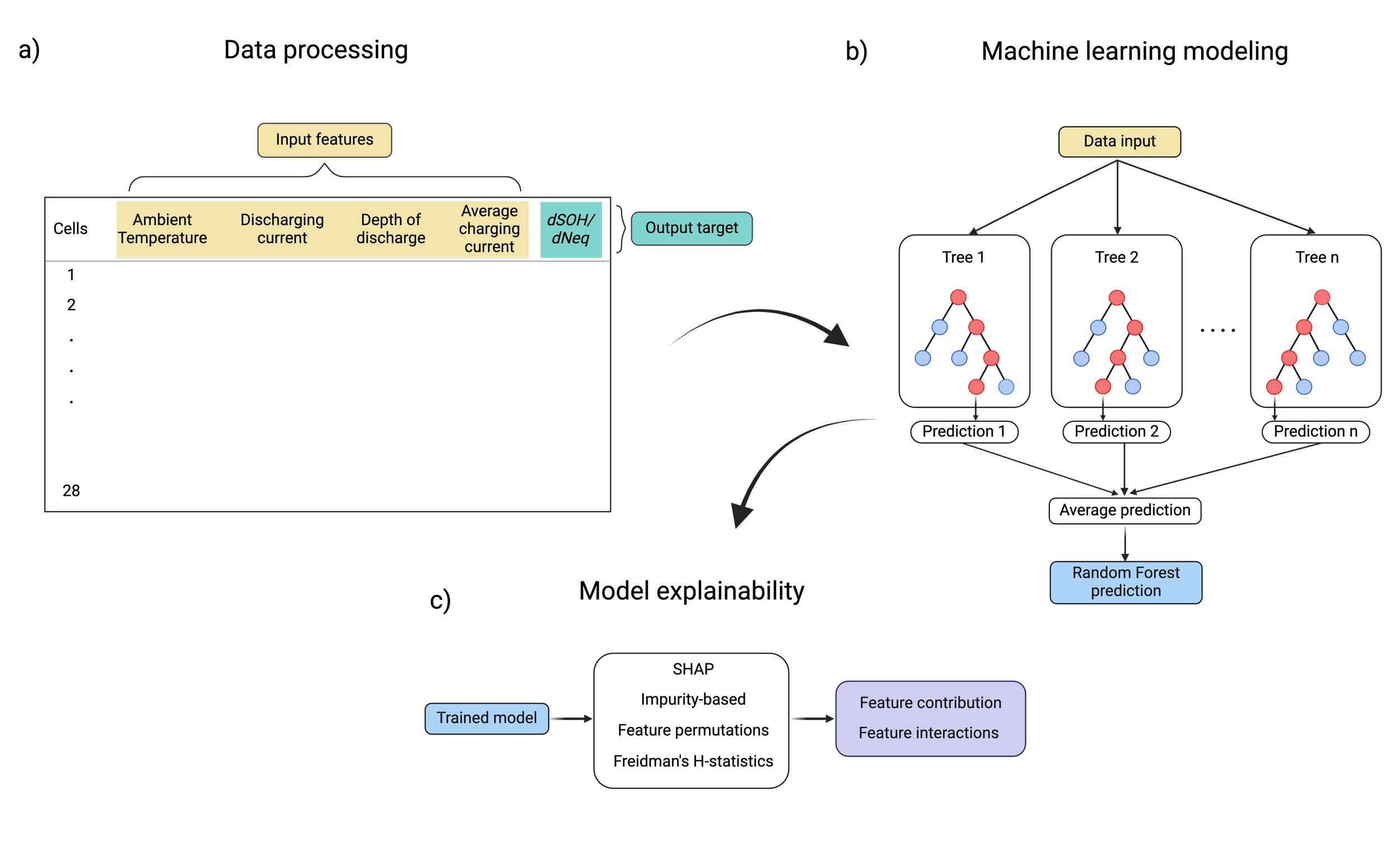